The rise of big data policing
A towering wall of computer screens blinks alive with crisis. A digital map of Los Angeles alerts to 911 calls. Television screens track breaking news stories.
Surveillance cameras monitor the streets. Rows of networked computers link analysts and police officers to a wealth of law enforcement intelligence.
Real-time crime data comes in. Real-time police deployments go out. This high-tech command center in downtown Los Angeles forecasts the future of policing in America.
Welcome to the Los Angeles Police Department’s Real-Time Analysis Critical Response (RACR) Division. The RACR Division, in partnership with Palantir—a private technology company that began developing social network software to track terrorists—has jumped head first into the big data age of policing.
Just as in the hunt for international terror networks, Palantir’s software system integrates, analyzes, and shares otherwise-hidden clues from a multitude of ordinary law enforcement data sources. A detective investigating a robbery suspect types a first name and a physical description into the computer—two fragmented clues that would have remained paper scraps of unusable data in an earlier era. The database searches for possible suspects.
Age, description, address, tattoos, gang affiliations, vehicle ownership instantly pop up in sortable fields. By matching known attributes, the computer narrows the search to a few choices. A photograph of a possible suspect’s car is identified from an automated license-plate reader scouring the city for data about
the location of every vehicle. Detectives follow up with a witness to identify the car used in the robbery. A match leads to an arrest and a closed case.
Roll call. Monday morning. Patrol officers receive digital maps of today’s “crime forecast.” Small red boxes signify areas of predicted crime. These boxes represent algorithmic forecasts of heightened criminal activity: years of accumulated crime data crunched by powerful computers to target precise city blocks. Informed by the data, “predictive policing” patrols will give additional attention to these “hot” areas during the shift.
Every day, police wait in the predicted locations looking for the forecast crime. The theory: put police in the box at the right time and stop a crime. The goal: to deter the criminal actors from victimizing that location.
Soon, real-time facial-recognition software will link existing video surveillance cameras and massive biometric databases to automatically identify people with open warrants. Soon, social media feeds will alert police to imminent violence from rival gangs.
Soon, data-matching technologies will find suspicious activity from billions of otherwise-anonymous consumer transactions and personal communications. By digitizing faces, communications, and patterns, police will instantly and accurately be able to investigate billions of all-too-human clues.
This is the future. This is the present. This is the beginning of big data policing.
At the center of policing’s future is data: crime data, personal data, gang data, associational data, locational data, environmental data, and a growing web of sensor and surveillance sources. This big data arises from the expanded ability to collect, store, sort, and analyze digital clues about crime.
Crime statistics are mined for patterns, and victims of violence are mapped in social networks. While video cameras watch our movements, private consumer data brokers map our interests and sell that information to law enforcement.
Phone numbers, emails, and finances can all be studied for suspicious links. Government agencies collect health, educational, and criminal records. Detectives monitor public Facebook, YouTube, and Twitter feeds. Aggregating data centers sort and study the accumulated information in local and federally funded fusion centers.
This is the big data world of law enforcement—still largely in its infancy but offering vastly more incriminating bits of data to use and study.
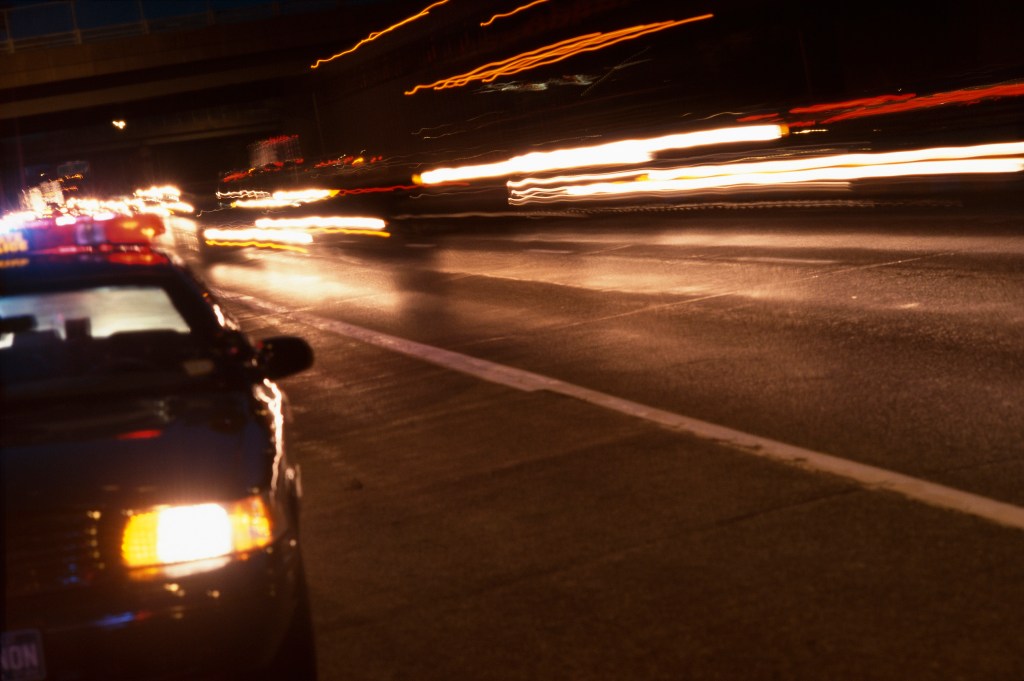
Photo: Jill Waterman/Photolibrary/Getty Images
Behind the data is technology: algorithms, network analysis, data mining, machine learning, and a host of computer technologies being refined and improved every day. Police can identify the street corner most likely to see the next car theft or the people most likely to be shot.
Prosecutors can target the crime networks most likely to destabilize communities, while analysts can link suspicious behaviors for further investigation. The decisional work of identifying criminal actors, networks, and patterns now starts with powerful computers crunching large data sets almost instantaneously. Math provides the muscle to prevent and prosecute crime.
Underneath the data and technology are people—individuals living their lives. Some of these people engage in crime, some not. Some live in poverty, some not. But all now find themselves encircled by big data’s reach. The math behind big data policing targets crime, but in many cities, crime suppression targets communities of color.
Data-driven policing means aggressive police presence, surveillance, and perceived harassment in those communities. Each data point translates to real human experience, and many times those experiences remain fraught with all-too-human bias, fear, distrust, and racial tension. For those communities, especially poor communities of color, these data-collection efforts cast a dark shadow on the future.
These new technologies – innovations that make up the constellation of big data policing – will impact the “who,” “where,” “when,” and “how” we police. A race is on to transform policing. New developments in consumer data collection have merged with law enforcement’s desire to embrace “smart policing” principles in an effort to increase efficiency amid decreasing budgets. Data-driven technology offers a double win—do more with less resources, and do so in a seemingly objective and neutral manner. […]
The promise of “smarter” law enforcement is unquestionably real, but so is the fear of totalizing surveillance. Growing “law and order” rhetoric can lead to surveillance overreach. Police administrators, advocates, communities, and governments must confront those concerns before — not after — the technology’s implementation.

Photo: Mitchell Funk/Getty Images
And society must confront those challenges informed by an understanding of how race has fractured and delegitimized the criminal justice system for many citizens. … People of color, immigrants, religious minorities, the poor, protesters, government critics, and many others who encounter aggressive police surveillance are at increased risk.
But so is everyone, because every one of us produces a detailed data trail that exposes personal details. This data—suctioned up, sold, and surveilled—can be wrong. The algorithmic correlations can be wrong. And if police act on that inaccurate data, lives and liberty can be lost. …
The big data policing revolution has arrived. The singular insight of this innovation is that data-driven predictive technologies can identify and forecast risk for the future. Risk identification is also the goal of this book — to forecast the potential problems of big data policing as it reshapes law enforcement.
Long-standing tensions surrounding race, secrecy, privacy, power, and freedom are given new life in digital form with the advent of big data analytics. New technologies will open up new opportunities for investigation and surveillance. The technological environment is rich with possibility but also danger.
This book seeks to initiate a conversation on the growth of these innovations, with the hope that by exposing and explaining the distorting effects of data-driven policing, society can plan for its big data future.
*An excerpt from the book The Rise of Big Data Policing: Surveillance, Race, and the Future of Law Enforcement (2017)
Featured Image: JIM WATSON/AFP/Getty Images